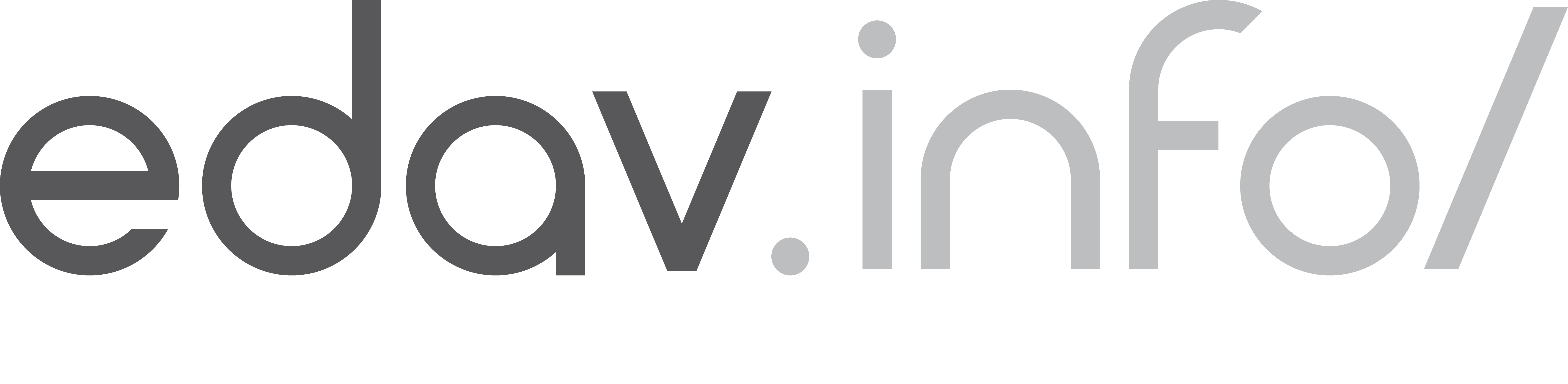
18 Interactive Geographic Data
This chapter originated as a community contribution created by AkhilPunia
This page is a work in progress. We appreciate any input you may have. If you would like to help improve this page, consider contributing to our repo.
18.1 Overview
You would have already seen different libraries that can help one in beautifully displaying geographic data like ggmap
and choroplethr
. Even though these libraries provide lots of interesting features to better express information through 2-dimensional graphs, they still lack one feature: interactivity. Here comes leaflet
—a library written in javascript to handle interactive maps. Fun Fact: It’s actively used by a lot of leading newspapers like The New York Times and The Washington Post.
Let’s dive in.
18.2 Brief Description about Dataset
For our analysis, we are using NYC Open Data about schools in New York City in 2016. You can find more about it on the Kaggle page. We will be focusing on the the distribution of different variables as a factor of geographical positions.
library(tidyverse)
library(leaflet)
library(htmltools)
library(leaflet.extras)
library(viridis)
schools <- read_csv('data/2016_school_explorer.csv')
18.3 Plotting Markers
Here we can see that all the Private schools in NYC have been plotted on a map that allows one to zoom in and out. The markers are used to denote the location of each individual school. If we hover over a marker, it displays the name of the school. Isn’t that cool!
Here’s the code for it:
lat<-median(schools$Latitude)
lon<-median(schools$Longitude)
schools %>%
filter(`Community School?`=="Yes") %>%
leaflet(options = leafletOptions(dragging = TRUE)) %>%
addTiles() %>%
addMarkers(label=~`School Name`) %>%
setView(lng=lon,lat=lat, zoom = 10)
18.4 Dynamic Heatmaps
Heatmaps are really useful tools for visualizing the distribution of a particular variable over a certain region (they are so useful, we got a page on ’em). In this example, we see how leaflet
is able to dynamically calculate the number of schools in a given region from just latitude and longitude data. You can experience this by zooming in and out of the graph.
Here’s the code for it:
lat<-mean(schools$Latitude)
lon<-mean(schools$Longitude)
leaflet(schools) %>%
addProviderTiles(providers$CartoDB.DarkMatterNoLabels) %>%
addWebGLHeatmap(size=15,units="px") %>%
setView(lng=lon,lat=lat, zoom = 10)
18.5 Dynamic Clustering
Here we can see how leaflet
allows one to dynamically cluster data based on its geographic distance at a given zoom level.
Here’s the code for it:
schools %>%
leaflet() %>%
addTiles() %>%
addCircleMarkers(radius = 2, label = ~htmlEscape(`School Name`),
clusterOptions = markerClusterOptions())
18.6 Plotting Groups
Here’s the code for it:
top<- schools %>%
group_by(District)%>%
summarise(top=length(unique(`School Name`)),lon=mean(Longitude),lat=mean(Latitude))%>%
arrange(desc(top))%>%
head(10)
pal <- colorFactor(viridis(100),levels=top$District )
top %>%
leaflet(options = leafletOptions(dragging = TRUE)) %>%
addProviderTiles(providers$CartoDB.DarkMatterNoLabels) %>%
addCircleMarkers(radius=~top/10,label=~paste0("District ", District," - ", top," Schools"),color=~pal(District),opacity = 1) %>%
setView(lng=lon,lat=lat, zoom = 10) %>%
addLegend("topright", pal = pal,
values = ~District,
title = "District",
opacity = 0.8)
18.7 Plotting Categorical Data
We can visualize the distribution of a particular class over the common map. This is achieved through an interactive widget provided on the top right that allows one to choose a particular category or multiple categories. The example below explores how schools in different neighborhoods are racially segregated.
Here’s the code for it:
ss <- schools %>% dplyr::select(`School Name`,Latitude, Longitude,`Percent White`, `Percent Black`, `Percent Asian`, `Percent Hispanic`)
segregation <- function(x){
majority = c()
w <- gsub("%","",x$`Percent White`)
b <- gsub("%","",x$`Percent Black`)
a <- gsub("%","",x$`Percent Asian`)
h <- gsub("%","",x$`Percent Hispanic`)
for (i in seq(1,nrow(ss))){
if (max(w[i],b[i],a[i],h[i]) == w[i])
{majority <- c(majority,'White')}
else if (max(w[i],b[i],a[i],h[i]) == b[i])
{majority <- c(majority,'Black')}
else if (max(w[i],b[i],a[i],h[i]) == a[i])
{majority <- c(majority,'Asian')}
else if (max(w[i],b[i],a[i],h[i]) == h[i])
{majority <- c(majority,'Hispanic')}
}
return(majority)
}
ss$race <- segregation(ss)
white <- ss %>% filter(race == "White")
black <- ss %>% filter(race == "Black")
hispanic <- ss %>% filter(race == "Hispanic")
asian <- ss %>% filter(race =="Asian")
lng <- median(ss$Longitude)
lat <- median(ss$Latitude)
pal_sector <- colorFactor( viridis(4), levels = ss$race)
m3 <- leaflet() %>% addProviderTiles("CartoDB") %>%
addCircleMarkers(data = white, radius = 2, label = ~htmlEscape(`School Name`),
color = ~pal_sector(race), group = "White")
m3 %>% addCircleMarkers(data = black, radius = 2, label = ~htmlEscape(`School Name`),
color = ~pal_sector(race), group = "Black") %>%
addCircleMarkers(data = hispanic, radius = 2, label = ~htmlEscape(`School Name`),
color = ~pal_sector(race), group = "Hispanic") %>%
addCircleMarkers(data = asian, radius = 2, label = ~htmlEscape(`School Name`),
color = ~pal_sector(race), group = "Asian") %>%
addLayersControl(overlayGroups = c("White", "Black","Hispanic","Asian")) %>%
setView(lng=lng,lat=lat,zoom=10)
These examples provide only a glimpse to what is truly possible with this library. If you want to explore more features and use-cases, check out the links listed below.
18.8 External Resources
- Leaflet in R Documentation: main documentation of the package.
- Basic leaflet maps in R: tutorial with examples.
- Interesting Kaggle Kernel visualizing earthquake data using leaflet in R: another use-case to explore.
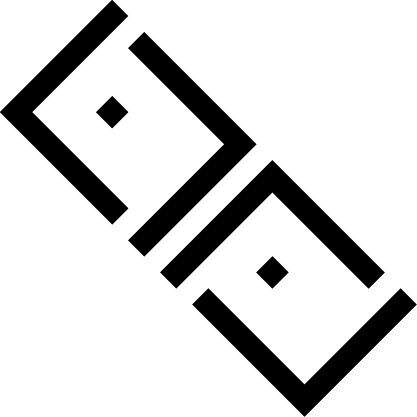
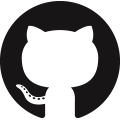
with